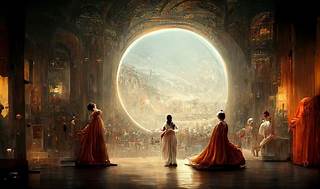
Explore
The above image was created by CoPilot using their generative artificial intelligence (generative AI) engine. GenAI, or GAI)refers to models or algorithms that create brand-new output, such as text, photos, videos, code, data, or 3D renderings, from the vast amount of data they are trained on. These models “generate” new content by referring to their training data, making novel predictions. In essence, generative AI learns patterns and structures from existing data and then produces new data with similar characteristics. It’s a fascinating field with applications ranging from text and image generation to protein design.
Generative artificial intelligence (generative AI, GenAI, or GAI) refers to models or algorithms that create brand-new output, such as text, photos, videos, code, data, or 3D renderings, from the vast amount of data they are trained on. These models “generate” new content by referring to their training data, making novel predictions. In essence, generative AI learns patterns and structures from existing data and then produces new data with similar characteristics. It’s a fascinating field with applications ranging from text and image generation to protein design.
Generative AI and traditional machine learning differ in their core focus and capabilities:
1. Generative AI:
– Focus: Generative AI models are designed to create new data samples autonomously. They learn patterns from existing data and then generate novel content that resembles the training data.
– Applications:
– Image Synthesis: Generative AI can create realistic images that don’t exist in the real world, making it useful for graphic design and entertainment.
– Text Generation: It can craft human-like text, handy for chatbots and language translation.
– Drug Discovery: By understanding known drug patterns, it suggests new molecules for faster drug development¹.
– Example: Imagine an AI artist creating unique digital paintings or generating new music compositions.
2. Traditional Machine Learning:
– Focus: Traditional ML primarily concerns learning patterns and making predictions based on existing data. It doesn’t explicitly generate new data.
– Algorithms: It includes supervised learning, unsupervised learning, and reinforcement learning.
– Example: Predicting house prices based on historical data or classifying spam emails.
In summary, generative AI goes beyond prediction, tapping into creativity by autonomously generating fresh content, while traditional ML focuses on analyzing existing data for decision-making.